Interactive explorer of origin-destination matrices: a Shiny app and how-to
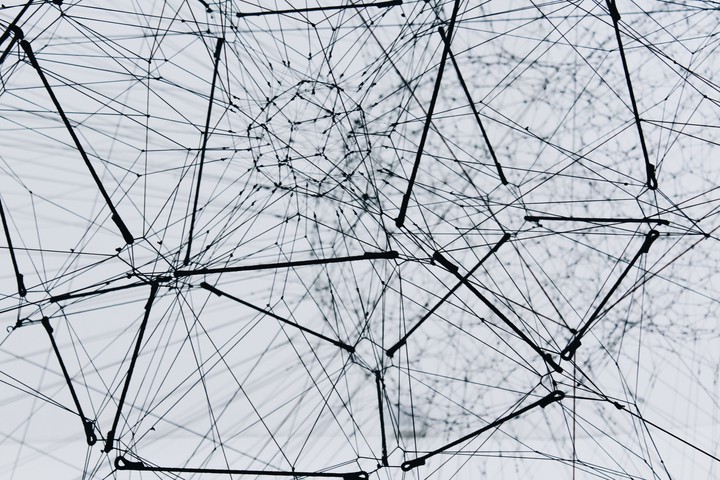
Interactive ODM explorer 1
Below is a Shiny app where you can interactively explore origin-destination (OD) matrices based on the travel survey of the Netherlands (2017).
It is featured with
- OD matrices broken down by mode (Non-bike, bike, and e-bike) and time (0 - 23)
- Interactive visualization of the networks
- Data come from North Holland which is known by its popularity of biking
Data preparation
With travel surveys, the first thing to do is to convert travel survey trip records into origin-destination pairs with a zoning system. A typical OD flow dataset looks like the below.
Origin zone | Destination zone | Trip number |
---|---|---|
100 | 100 | 45 |
101 | 100 | 78 |
100 | 101 | 22 |
101 | 114 | 1 |
After making the zone id just like the zoning system, there is a great tutorial on how to generate OD Polylines with the origin and destination of each connection consistent with their polygons' centroid.
A Shiny app
Shiny is a web application framework for R. It allows you to quickly create your web-based data product. You can create one inside RStudio and fill in the below two scripts.
ui.R
Below is the skeleton of the script for clarity. A tutorial for beginners of Shiny layout can be found here. More detailed description of the control widgets can be found here.
library(shiny)
library(dplyr)
library(sf)
library(stplanr)
library(tmap)
# Define UI for application that draws a OD network
shinyUI(fixedPage(
# Application title
titlePanel("Origin-destination matrices (ODMs) explorer"),
sidebarLayout(
sidebarPanel(
radioButtons("mode", ...),
radioButtons("time", ...),
h5("No will show the results of all trips over 24 hours."),
hr(),
conditionalPanel("input.time == 1",...),
submitButton("Plot"),
...
),
mainPanel(
...,
plotOutput("netPlot", height = "800px", width="100%"),
...
)
)
))
submitButton
is particularly useful when the data size is big. Prepare input and hit the plot button to see the results.server.R
We now fill in what to do with the input
to produce the output
inside shinyServer(function(input, output){...})
. All the loaded libraries and created variables in ui.R and server.R are sharing the same environment.
data/file2use.csv
instead of ../../data/file2use.csv
.1 Load OD data and preprocess
The first step inside shinyServer(function(input, output){...})
is to load data and preprocess.
df <- read.csv('data/odms_by_mode.csv')
zones <- st_transform(st_read('data/zones_subset.shp'), crs = 4326)
colnames(df) <- c("ozone", "dzone", "Non-bike", "E-bike", "Bike", "Total", 'Hour')
df <- df %>% filter(ozone %in% zones$zone, dzone %in% zones$zone)
2 Prepare data based input in ui.R
Taking all the input, mode
, time
, and hour
, the below chunk subsets the data and creates Polylines using the R package stplanr
.
odm <- reactive({
# Subset data based on input$mode and input$hour from ui.R
md <- input$mode
hr <- ifelse(input$time == 0, 24, input$hour)
df_a <- df %>%
select(c('ozone', 'dzone', !!md, 'Total', 'Hour')) %>%
filter(ozone != dzone,
Hour == hr) %>%
mutate(trip = get(md) / sum(get(md)) * 100) %>%
filter(trip > 0) %>%
mutate(share = get(md) / Total * 100)
# Create lines between OD zones
lines <- od2line(flow = df_a, zones = zones)
lines <- arrange(lines, trip)
return(lines)
})
odm
is a function to be called later.3 Prepare data based input in ui.R
Finally, we are ready to plot the OD network using R package tmap
.
output$netPlot <- renderPlot({
# draw the ODMs
tm_shape(zones) +
tm_fill(col="grey35") +
tm_borders("white", alpha=.2) +
tm_shape(odm()) +
tm_lines(
palette = "plasma",
trans = "log10", style="cont",
lwd = "trip",
scale = 9,
title.lwd = 0.5,
alpha = 0.5,
col = "share",
title = "Mode share (%)",
legend.lwd.show = FALSE
) +
tm_scale_bar() +
tm_layout(
title = input$mode,
frame = FALSE,
legend.bg.alpha = 0.5,
legend.bg.color = "white"
)
})
Source code on GitHub.
I’ve been taking Data Science Specialization on Coursera. BTW, it is a well-structured specialization. Highly recommended! This post is based on the final project of Course 9/10 Developing Data Products. ↩︎